The relevance of data today is well known: it fuels emerging technologies such as Artificial Intelligence or Machine Learning, improves decision making, generates ultra-targeted advertising, and so on. Thus, 78% of IT decision makers agree that data collection and analysis has the potential to change the way their company does business in the next 1-3 years.
However, data processing has a major drawback, namely the exposure of users’ privacy. To avoid this evil, regulations such as the GDPR impose the protection of personal data as a maxim on data controllers.
The most recent studies show that companies have made significant efforts to safeguard data privacy, although these have not been sufficient. In 2021, 95% of business leaders reported having strong or very strong data protection measures in place, but 62% agreed that their companies should do more. From the users’ perspective, the results are no more encouraging, given that they do not trust that they are guaranteed real protection. Eighty-six per cent said they had growing concerns about data privacy, with around half fearing that their data could be hacked (51%) or sold (47%).
The truth is that there are still some gaps in providing maximum protection for data processing. This is the case, for example, with data analytics and data sharing. Unlike when they are stored or transmitted, data sets are exposed when they are manipulated, as their use has so far been unfeasible with solutions such as encryption.
Thus, one of the main challenges facing companies right now is how to perform data analysis while protecting this data and respecting the privacy requests of the individuals whose data it concerns. It is in this context that the recent strategic categorisation of so-called Privacy-Enhancing Computations (PECs), a set of technologies that allow data to be analysed and shared without exposing its content to third parties, thus securing the data while it is being used, has to be understood.
Current situation of PECs
PECs have been applied in the public and academic sector for years. Originally, they referred to a group of relatively simple technologies related to information masking, such as anonymisation or pseudonymisation techniques, which avoid the identification of the subjects concerned.
Earlier techniques, on the other hand, were not entirely effective; for example, by combining them with additional datasets, reconstruction of the original database can be carried out, with the possibility of re-identifying the subjects. Now, however, with the growing interest, PECs are reaching the level of refinement necessary to meet the level of demand required by companies. This branch of technology is currently undergoing a very high rate of development, above the average rate of improvement of other technologies. According to data from the Massachusetts Institute of Technology’s search portal, innovation in PEC is growing at 178% per year, second only to cloud computing technology.
Annual rate of technological innovation
Estimated annual improvement by 2022
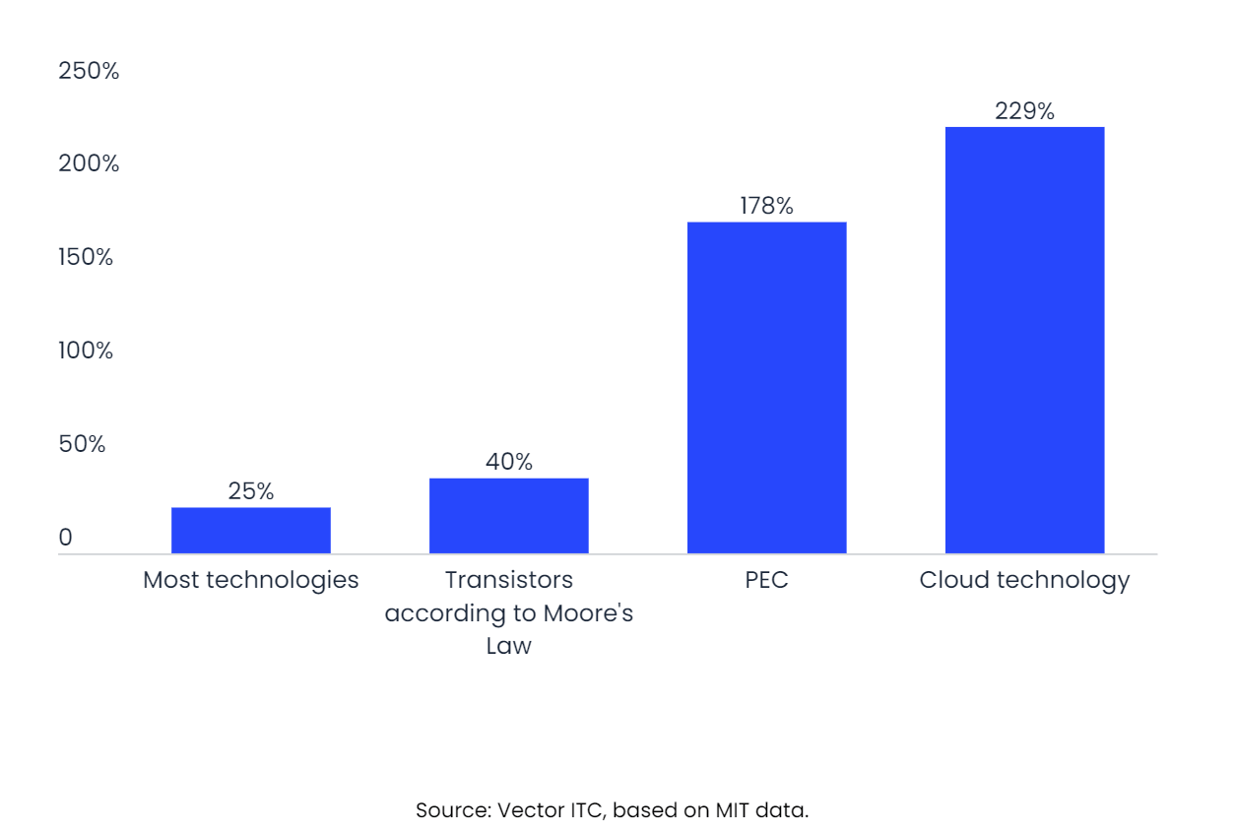
As a result of recent advances in privacy enhancements, new, more sophisticated and effective PEC technologies have emerged and are now gaining attention and beginning to be applied to practical projects. A recent World Economic Forum report identifies and differentiates 5 emerging PEC techniques:
- Homomorphic encryption. This is an encryption method that allows computational operations to be performed on encrypted data. In this way, when analytical operations are performed, an encrypted result is generated which, when decrypted, matches the result of the operations as if they had been performed on unencrypted data. It is useful for sharing data for analytical purposes, as it remains unreadable, but operable.
- Secure multi-part computation. This is a cryptographic technique that is actually a sub-type of the previous one. Its particularity is to allow the computation of values from multiple encrypted data sources, thus allowing for greater complexity. It is ideal for creating an environment of trust in which different institutions share personal data without compromising privacy.
- Federated analytics. This is a new technology that enables the execution of in situ analysis, i.e. performed on the raw data that is stored locally on the devices that collect it. With this possibility in hand, it is not necessary to centrally collect the collected data, but instead, aggregated results can be provided to the subject or ingenuity that requires them, ensuring that the data never leave the device that generated them. This innovation is closely linked to federated learning, in which local devices such as smartphones have the capacity to train predictive models and share their results, weaving a collaborative network to jointly improve the application together with other users.
- Zero-knowledge testing. This is a technological innovation that makes it possible to validate that a piece of information is true without the need to expose the data that proves it. This is possible thanks to a series of cryptographic algorithms by means of which a ‘tester’ can mathematically prove to a ‘verifier’ that a computational statement is correct and without the need for any intermediary. This satisfies the principle of information minimisation, and is useful for accessing services through the accreditation of personal data.
- Differential privacy. This is another CSP that intervenes in data sets by introducing a layer of ‘random noise’ that prevents specific data about each individual piece of information from being known, without changing the end result. In a manner of speaking, it generates an alternative, but identical, database, thus ensuring that group patterns are described while maintaining the privacy of individuals. Again, it is a practical method for securely sharing data for analytical purposes.
Forward-looking view of the trend
Judging by the pace of innovation of the PECs, the emerging techniques described above will consolidate their degree of perfection, and the introduction of some other even more complex and effective novelty cannot be ruled out.
For companies, their adoption will lead to a substantial improvement in data protection, since, as indicated above, they focus on the analytical part, when data is most exposed, and to which a satisfactory solution has not yet been found. This will lead to two potential benefits:
- Stricter compliance with data protection regulations, which in turn results in avoiding the financial losses generated by fines for non-compliance. The various breaches committed since the entry into force of the new GDPR regime in May 2018 until January 2021, amounting to around $332.4 million in fines according to the law firm DLA Piper, reveal both the lack of adjustment of European companies to the regulation and the high economic cost that this entails for them.
- A reduction in leaks of personal data, which will prevent more scandals or reputational risks for companies and which, for example, can lead to loss of alliances or consumer distrust. In general, better data protection will help to make users more willing to share data.
Furthermore, the incorporation of techniques such as differential privacy or homomorphic encryption and its subcategory, multiparty computation, provides the opportunity to share data sets and allow other parties to operate on them without exposing their content. Precisely one of the biggest risks in the relationship with third parties is the breach of data privacy. Studies such as the one by Forrester indicate that the costs derived from a data breach increase by an average of 370,000 dollars when caused by a third party. Implementing these innovations will therefore mean working securely in multiple, untrusted environments, consolidating three current practices:
- Sharing databases with external providers for application testing and the execution of the required analytics, when the organisation itself does not have sufficient capacity to do so.
- Sectoral collaboration in the exchange of data. This is what is known as second-party data, first-party data that other companies are willing to share, increasing the volume of information available to companies. This practice will become increasingly relevant as the removal of third-party cookies becomes more widespread and access to data provided by external providers becomes more restricted. As of 2021, around 75% of executives in the US and UK said their organisation was already sharing first-party data for insights, activations, measurement and attribution, or planned to do so, according to a report by Winterberry Group.
- Cooperation in the fight against fraud and crime financing. A key part of this fight is data sharing, as criminals often spread their activity across different institutions to make it difficult to trace their actions. In a secure environment, data can be shared seamlessly and without suspicion, combining the forces needed to put an end to this scourge.
Finally, decentralised ingenuities such as federated analytics will reduce companies’ internal access to the data they generate. Others, such as zero-knowledge testing, will minimise the information provided, but without losing its value. This will mean companies with the same or greater capacity to collect and analyse information, but with less awareness and depth of individual user data. Combined with the above technologies, the result is a context in which the value of data is maximised, while it is kept hidden from those who handle it, whether they are the data controllers or their partners.
Because of the improved protection and the other potential benefits, some reports estimate that the adoption of emerging PEC techniques will be rapid: by 2025, 50% of large enterprises will adopt PECs to securely process their data.
Examples of companies that are applying the new PEC techniques
There are a number of large companies that are investing in and starting to apply the PEC techniques described above. Some of them are:
- In early 2020, IBM Security was working with Banco Bradesco and other financial institutions to develop pilot tests of homomorphic encryption. Now, IBM Security has taken the next step and extended its product to a wider audience. Its new homomorphic encryption services provide a scalable hosting environment on IBM Cloud, along with consulting and administrative services to assist customers in learning and prototyping solutions that can leverage full homomorphic encryption.
- Alibaba Group and Cape Privacy, among others, are investing in and awaiting implementation of TF Encrypted. This is still experimental software, and has been developed by GitHub and other parties with the aim of being a framework for machine learning encryption in TensorFlow. It looks and feels like TensorFlow, leveraging the ease of use of the Keras API while enabling training and prediction on encrypted data through secure multi-part computation and homomorphic encryption.
- The giant Amazon employs a differential privacy model that its own research group developed. It does so to provide users’ personalised shopping preferences while covering sensitive information about their past purchases. In addition, the Amazon Science team is refining the technique to cover textual data analysis, which would work by rephrasing the text provided by the customer and basing the analysis on the new wording, rather than on the customers’ own language.
- Google is a pioneer in federated learning and consequently also in federated analytics. It has been applying it for 5 years to its GBoard keyboard on Android mobile phones. When Gboard displays a suggested query, the smartphone stores locally information about the current context and whether you clicked on the suggestion. Federated learning processes that history on the device to suggest improvements for the next iteration of Gboard’s query suggestion model. In this way, data stored on the mobile device is processed on the mobile device, and its analytics allow the app to be trained locally, without the need to have shared that information in the server cloud, thus ensuring the privacy of that data.
- Electronic Coin Company developed in 2016 the cryptocurrency known as Zcash, which enjoys complete anonymity in transactions on the Blockchain network. This has to do with the fact that its operations are carried out under zero-knowledge codes activated by algorithms of the zk-SNARK type, which allows the sender to validate a given transaction, the recipient and its amount before the network without revealing any kind of information. For this reason, Zcash transactions are fast, secure and confidential, and operate with low transaction costs of 0.0001 Zcash.
Conclusions
It can be said that PECs have undergone a substantial evolution in a short period of time. A number of emerging techniques have been added, displacing the original privacy-enhancing techniques, which hardly offered a satisfactory answer. The new ones, on the contrary, offer more optimised protection with various kinds of solutions: analysis on encrypted data, generation of a trusted environment for data sharing, and decentralised analysis.
The picture that opens up for the new PECs is a context in which the value of data is maximised, while it is kept hidden from data handlers and their partners, so that companies are getting closer and closer to analysing all kinds of data without violating users’ privacy.
The next step is the adoption of this set of innovations, which is happening now in large companies, which are testing and refining their own models. In five years’ time, probably more than half will have fully integrated them into their Big Data processes.